Features
“Autonomous driving is coming, for real this time”
By Igal Raichelgauz, visionary founder and CEO of Autobrains
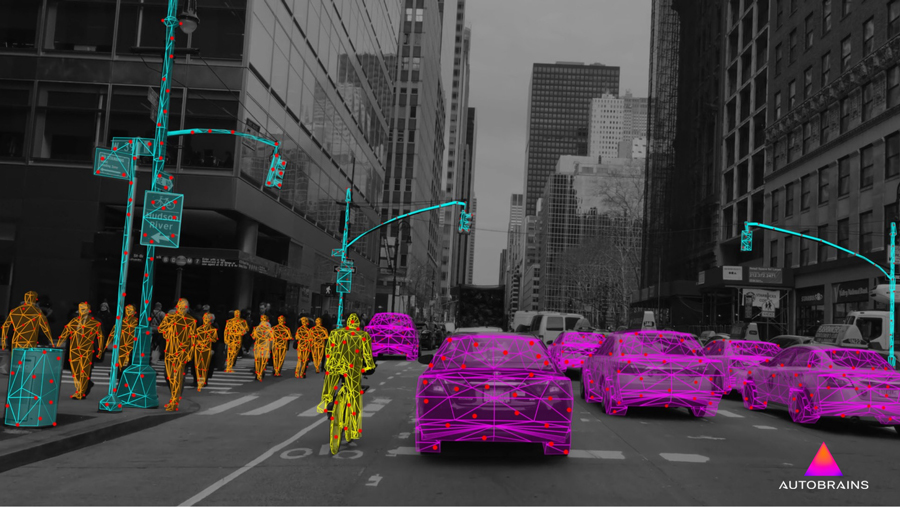
Equipped with billions in funding, numerous start-ups have tried to help autonomous driving achieve a breakthrough over the past ten years. So far, however, success has been modest and a long way from autonomy for all.
When people do drive autonomously, it is robotaxis that are used by companies such as Waymo and Cruise. They offer their service in predefined regions and rely on expensive sensors and computing resources, which are prohibitively expensive for vehicles outside of professionally operated fleets. Other companies, such as Mobileye and Momenta, focus on advanced driver assistance systems (ADAS), rarely beyond Level 3. Tesla is an exception; Elon Musk has asserted multiple times over the years that Tesla is on the brink of autonomous driving (AD) even if this promise has not yet been kept and autonomous Tesla cabs are to come first.
While the history of Tesla and autonomous driving has seen many setbacks and delays, I expect Tesla’s continued progress to motivate a new generation of AD startups, which I refer to as AD2.0, to keep working hard on the technology. This new wave includes companies like Wayve. Established players such as Cruise, which continues to have billions at its disposal for its robotaxi programs, are also continuing to get involved. Additionally, companies like Mobileye, Momenta and my own company, Autobrains, are scaling their offerings from ADAS to AD.
But the question remains: What will it take for AD2.0 companies to succeed where AD1.0 failed? The answer is three-part: technology, trust and Tesla.
1. Technology
- AD1.0’s approach was based on a “video game” concept. The belief was that if we could map the real world into a highly accurate 3D model, selfdriving would work similarly to how computers play video games and AI could drive better than humans.
To achieve this, the perfection of the perception module was optimized with the help of deep learning and vehicles were equipped with various sensors such as radar and lidar. Tesla is going its own way and has been limiting its sensors to cameras (analogous to human vision) for years. This is because the assumption is that non-human-like sensors, such as radar or lidar, would postpone the solution to the AI problem rather than solve it. Other players are continuing down the path of high-level technologization, adding further means (such as HD maps) to the AD system to compensate for the shortcomings in modeling the road environment in real time.
The “video game” approach of AD1.0 was not successful because detailed 3D models are not sufficient for driving in the real world. The human brain does not generate a 3D model of the world either. Instead, we manage partial data very well in ‘fuzzy’ and ‘unclear’ environments because we concentrate on what is necessary for certain actions in certain scenarios.
A year ago, Tesla began transitioning from the modular “video game” approach to the end-to-end approach. Instead of breaking the autonomous driving problem into perception models that serve as input to a decisioning model, the end-to-end approach uses a single giant neural network to address the entire problem. Elon Musk described it as “images in” and “steering, acceleration and braking out.” The basis for this transition is Tesla’s already collected large amount of data, training infrastructure and inference chips. Tesla has shown improvements in its Full Self-Driving (FSD) system, and other OEMs and startups are following suit.
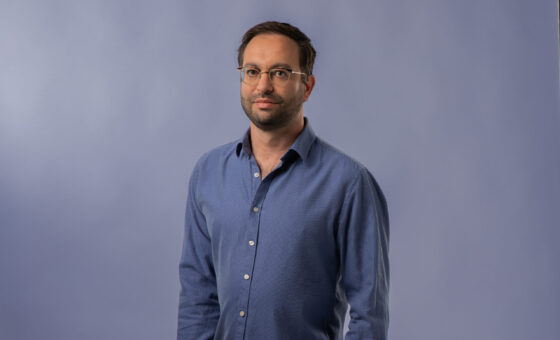
While the end-to-end approach is a step in the right direction, it remains a partial solution. The key issue is the reliance on a single AI network for real-world intelligence. This is because scaling to the enormous number of edge cases requires exponential resources, both in terms of computing power and data.
AD2.0 initiates the necessary change in thinking and leads away from a single end-to-end neural network. AD2.0 leads to a large-scale ensemble of end-to-end neural networks and moves away from learning and optimizing for the average case towards the continuous learning of new driving skills.
2. Trust
Creating consumer trust in AD also requires a massive rethink. After all, trust or credibility only comes from consistent, predictable and safe performance that is communicated to drivers in a transparent way. AD1.0 was not able to do this. AD2.0 takes a different approach and builds trust through predictability.
Necessary interventions by the driver without warning damage confidence in autonomous driving, even if they do not occur often. To build lasting trust, it will be crucial to predict interventions and inform the driver in advance.
Tesla’s current FSD performance comprises roughly one unexpected intervention every 10 trips. At Autobrains we believe the minimal required safety level for AD should be one unexpected incident every 50,000-100,000 trips. Paradoxically, as human intervention is needed less regularly, a new problem arises: “automation complacency,” where the driver becomes lax about supervising the AD system.
To build trust, AD2.0 will split driving into two modes: scenarios for autonomous driving (unsupervised mode), where you can trust the car, and scenarios where the driver must supervise the car (supervised mode).
For unsupervised, i.e., autonomous, operation, we at Autobrains believe that the minimum target for safe driving should be more than 100,000 trips per unexpected event. For supervised mode, a higher number of driver interventions is acceptable. We believe that our AI technology can achieve safe AD for over 99 percent of driving time by 2027. The small remainder will need to be monitored by the driver. However, we believe in the ability of our AI to cover emerging edge cases, which will continuously reduce the monitored driving time.
The most important capability of the AI in this case is the announcement of the type of intervention required. This will become possible by breaking the AD problem into a large (but finite) set of driving skills, each one separately learned, matured, evaluated and incorporated into the car.
3. Tesla
For the reasons mentioned above, I expect Tesla to lead the autonomous driving revolution. Tesla’s cars and strategy are designed for autonomous driving, and the recently announced Robotaxi is proof of this. The expected success of FSD will have a direct impact on stock performance. Tesla could become the most valuable company in the world if it achieves true autonomy. However, if the company misses its milestones, Tesla’s importance could be reduced to that of a normal car manufacturer.
Final Thoughts
I am convinced that AD2.0 will work, through a combination of technological advances in our AI technology, an innovative approach to gaining consumer trust, and Tesla’s role as a trailblazer.

모빌리티(Mobility)의 미래 비즈니스 전략을 찾다
- 모빌리티타임즈 (mobilitytimes.net)
-
Features2개월 ago
중국 로봇 개에 대한 국가안보 위협 시나리오.. 미 하원 특위, 유니트리 로보틱스 전면금지 촉구
-
News2개월 ago
오토폼엔지니어링, 한국서 ‘AI 기반 디지털 금형 생태계’ 구축 본격화
-
News2개월 ago
“카드 한 장이면 끝!”.. 에바씨엔피, 전기차주 위한 ‘꿀템 카드’ 출시
-
Policy2개월 ago
국토교통부, 창업기업 지원 강화… ‘국토교통 창업지원센터’ 입주기업 모집
-
Products1개월 ago
NXP, 2세대 차량용 개발 플랫폼 ‘오렌지박스 2.0’로 SDV 시대 지원
-
News1개월 ago
인피니언-한화 NxMD, 자동차 무선 커넥티비티 혁신 위한 전략적 제휴 체결
-
News1개월 ago
엘앤에프, ‘뉴로컬리즘’ 실천하는 대구발 이차전지 소재 기업
-
Products2개월 ago
넥스페리아, D2PAK-7 패키징의 업계 최고 자동차 인증 1200V SiC MOSFET 출시